Xarray 与 Dask Arrays
目录
在线 Notebook
您可以在一个在线会话中运行此 notebook ,或在 Github 上查看它。
Xarray 与 Dask Arrays¶
Xarray 是一个开源项目和 Python 包,它将 Pandas 的带标签数据功能扩展到 N 维数组类数据集。它与 NumPy 和 Pandas 共享相似的 API,并在底层支持 Dask 和 NumPy 数组。
[1]:
%matplotlib inline
from dask.distributed import Client
import xarray as xr
启动 Dask 客户端以查看仪表盘¶
启动 Dask 客户端是可选的。它将提供一个仪表盘,有助于了解计算情况。
在下方创建客户端后,仪表盘的链接将可见。我们建议您在使用 notebook 时,将仪表盘打开在屏幕一侧。调整窗口位置可能需要一些精力,但在学习时同时查看它们非常有用。
[2]:
client = Client(n_workers=2, threads_per_worker=2, memory_limit='1GB')
client
[2]:
客户端
Client-4eea680b-0de0-11ed-9d1a-000d3a8f7959
连接方法: Cluster object | 集群类型: distributed.LocalCluster |
仪表盘: http://127.0.0.1:8787/status |
集群信息
LocalCluster
8c9bb588
仪表盘: http://127.0.0.1:8787/status | 工作节点 2 |
总线程数 4 | 总内存: 1.86 GiB |
状态: 运行中 | 使用进程: True |
调度器信息
调度器
Scheduler-f26c7784-2ac7-471c-91ac-1b0f9e3135b1
通信: tcp://127.0.0.1:36327 | 工作节点 2 |
仪表盘: http://127.0.0.1:8787/status | 总线程数 4 |
启动时间: 刚刚 | 总内存: 1.86 GiB |
工作节点
工作节点: 0
通信: tcp://127.0.0.1:34819 | 总线程数 2 |
仪表盘: http://127.0.0.1:39963/status | 内存: 0.93 GiB |
Nanny: tcp://127.0.0.1:38683 | |
本地目录: /home/runner/work/dask-examples/dask-examples/dask-worker-space/worker-kg42o2xu |
工作节点: 1
通信: tcp://127.0.0.1:39355 | 总线程数 2 |
仪表盘: http://127.0.0.1:43051/status | 内存: 0.93 GiB |
Nanny: tcp://127.0.0.1:43647 | |
本地目录: /home/runner/work/dask-examples/dask-examples/dask-worker-space/worker-q4dslyjg |
打开一个示例数据集¶
在此示例中,我们将使用 xarray 的一些教程数据。通过指定分块形状,xarray 将自动为 Dataset
中的每个数据变量创建 Dask 数组。在 xarray 中,Datasets
是带标签数组的类似字典的容器,类似于 pandas.DataFrame`. 请注意,我们在指定分块形状时利用了 xarray 的维度标签。
[3]:
ds = xr.tutorial.open_dataset('air_temperature',
chunks={'lat': 25, 'lon': 25, 'time': -1})
ds
[3]:
<xarray.Dataset> Dimensions: (lat: 25, time: 2920, lon: 53) Coordinates: * lat (lat) float32 75.0 72.5 70.0 67.5 65.0 ... 25.0 22.5 20.0 17.5 15.0 * lon (lon) float32 200.0 202.5 205.0 207.5 ... 322.5 325.0 327.5 330.0 * time (time) datetime64[ns] 2013-01-01 ... 2014-12-31T18:00:00 Data variables: air (time, lat, lon) float32 dask.array<chunksize=(2920, 25, 25), meta=np.ndarray> Attributes: Conventions: COARDS title: 4x daily NMC reanalysis (1948) description: Data is from NMC initialized reanalysis\n(4x/day). These a... platform: Model references: http://www.esrl.noaa.gov/psd/data/gridded/data.ncep.reanaly...
快速检查上面的 Dataset
,我们会注意到此 Dataset
有三个维度,类似于 NumPy 中的轴(lat
、lon
和 time
),三个坐标变量,类似于 pandas.Index
对象(也命名为 lat
、lon
和 time
),以及一个数据变量(air
)。Xarray 还将 Dataset 特定的元数据作为属性保存。
[4]:
da = ds['air']
da
[4]:
<xarray.DataArray 'air' (time: 2920, lat: 25, lon: 53)> dask.array<open_dataset-ced301335a37488ca2d3a9447fa27157air, shape=(2920, 25, 53), dtype=float32, chunksize=(2920, 25, 25), chunktype=numpy.ndarray> Coordinates: * lat (lat) float32 75.0 72.5 70.0 67.5 65.0 ... 25.0 22.5 20.0 17.5 15.0 * lon (lon) float32 200.0 202.5 205.0 207.5 ... 322.5 325.0 327.5 330.0 * time (time) datetime64[ns] 2013-01-01 ... 2014-12-31T18:00:00 Attributes: long_name: 4xDaily Air temperature at sigma level 995 units: degK precision: 2 GRIB_id: 11 GRIB_name: TMP var_desc: Air temperature dataset: NMC Reanalysis level_desc: Surface statistic: Individual Obs parent_stat: Other actual_range: [185.16 322.1 ]
xarray 中的每个数据变量都称为 DataArray
。这些是 xarray 中基本的带标签数组对象。与 Dataset
非常相似,DataArrays
也具有支持其许多基于标签的操作的维度和坐标。
[5]:
da.data
[5]:
|
通过 data
属性可以访问底层数据数组。在这里我们可以看到我们有一个 Dask 数组。如果此数组由 NumPy 数组支持,则此属性将指向数组中的实际值。
使用标准的 Xarray 操作¶
几乎在所有情况下,无论底层数据存储为 Dask 数组还是 NumPy 数组,使用 xarray 对象的操作都是相同的。
[6]:
da2 = da.groupby('time.month').mean('time')
da3 = da - da2
da3
[6]:
<xarray.DataArray 'air' (time: 2920, lat: 25, lon: 53, month: 12)> dask.array<sub, shape=(2920, 25, 53, 12), dtype=float32, chunksize=(2920, 25, 25, 1), chunktype=numpy.ndarray> Coordinates: * lat (lat) float32 75.0 72.5 70.0 67.5 65.0 ... 25.0 22.5 20.0 17.5 15.0 * lon (lon) float32 200.0 202.5 205.0 207.5 ... 322.5 325.0 327.5 330.0 * time (time) datetime64[ns] 2013-01-01 ... 2014-12-31T18:00:00 * month (month) int64 1 2 3 4 5 6 7 8 9 10 11 12
当您希望将结果作为数据存储在 NumPy 数组中的 xarray.DataArray
时,调用 .compute()
或 .load()
。
如果您在上面启动了 Client()
,那么您可能想在计算期间查看状态页面。
[7]:
computed_da = da3.load()
type(computed_da.data)
[7]:
numpy.ndarray
[8]:
computed_da
[8]:
<xarray.DataArray 'air' (time: 2920, lat: 25, lon: 53, month: 12)> array([[[[-5.14987183e+00, -5.47715759e+00, -9.83168030e+00, ..., -2.06136017e+01, -1.25448456e+01, -6.77099609e+00], [-3.88607788e+00, -3.90576172e+00, -8.17987061e+00, ..., -1.87125549e+01, -1.11448669e+01, -5.52117920e+00], [-2.71517944e+00, -2.44839478e+00, -6.68945312e+00, ..., -1.70036011e+01, -9.99716187e+00, -4.41302490e+00], ..., [-1.02611389e+01, -9.05839539e+00, -9.39399719e+00, ..., -1.53933716e+01, -1.01606750e+01, -6.97190857e+00], [-8.58795166e+00, -7.50210571e+00, -7.61483765e+00, ..., -1.35699463e+01, -8.43449402e+00, -5.52383423e+00], [-7.04670715e+00, -5.84384155e+00, -5.70956421e+00, ..., -1.18162537e+01, -6.54209900e+00, -4.02824402e+00]], [[-5.05761719e+00, -4.00010681e+00, -9.17195129e+00, ..., -2.52222595e+01, -1.53296814e+01, -5.93362427e+00], [-4.40733337e+00, -3.25991821e+00, -8.36616516e+00, ..., -2.44294434e+01, -1.41292725e+01, -5.66036987e+00], [-4.01040649e+00, -2.77757263e+00, -7.87347412e+00, ..., -2.40147858e+01, -1.34914398e+01, -5.78581238e+00], ... -3.56890869e+00, -2.47412109e+00, -1.16558838e+00], [ 6.08795166e-01, 1.47219849e+00, 1.11965942e+00, ..., -3.59872437e+00, -2.50396729e+00, -1.15667725e+00], [ 6.59942627e-01, 1.48742676e+00, 1.03787231e+00, ..., -3.84628296e+00, -2.71829224e+00, -1.33132935e+00]], [[ 5.35827637e-01, 4.01092529e-01, 3.08258057e-01, ..., -1.68054199e+00, -1.12142944e+00, -1.90887451e-01], [ 8.51684570e-01, 8.73504639e-01, 6.26892090e-01, ..., -1.33462524e+00, -7.66601562e-01, 1.03210449e-01], [ 1.04107666e+00, 1.23202515e+00, 8.63311768e-01, ..., -1.06607056e+00, -5.31036377e-01, 3.14453125e-01], ..., [ 4.72015381e-01, 1.32940674e+00, 1.15509033e+00, ..., -3.23403931e+00, -2.23956299e+00, -1.11035156e+00], [ 4.14459229e-01, 1.23419189e+00, 1.07876587e+00, ..., -3.47311401e+00, -2.56188965e+00, -1.37548828e+00], [ 5.35278320e-02, 8.10333252e-01, 6.73461914e-01, ..., -4.07232666e+00, -3.12890625e+00, -1.84762573e+00]]]], dtype=float32) Coordinates: * lat (lat) float32 75.0 72.5 70.0 67.5 65.0 ... 25.0 22.5 20.0 17.5 15.0 * lon (lon) float32 200.0 202.5 205.0 207.5 ... 322.5 325.0 327.5 330.0 * time (time) datetime64[ns] 2013-01-01 ... 2014-12-31T18:00:00 * month (month) int64 1 2 3 4 5 6 7 8 9 10 11 12
时间序列操作¶
因为我们有一个 datetime 索引,时间序列操作可以高效工作。在这里我们演示 xarray 的 resample 方法的使用
[10]:
da.resample(time='1w').mean('time').std('time')
[10]:
<xarray.DataArray 'air' (lat: 25, lon: 53)> dask.array<_sqrt, shape=(25, 53), dtype=float32, chunksize=(25, 25), chunktype=numpy.ndarray> Coordinates: * lat (lat) float32 75.0 72.5 70.0 67.5 65.0 ... 25.0 22.5 20.0 17.5 15.0 * lon (lon) float32 200.0 202.5 205.0 207.5 ... 322.5 325.0 327.5 330.0
[11]:
da.resample(time='1w').mean('time').std('time').load().plot(figsize=(12, 8))
[11]:
<matplotlib.collections.QuadMesh at 0x7fc71443de80>
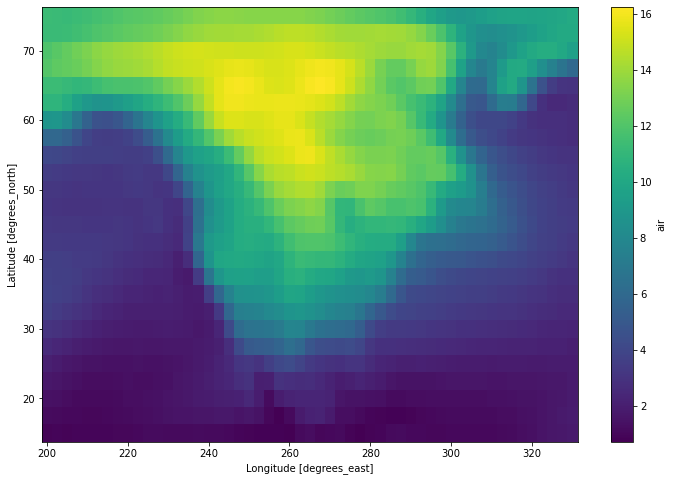
和滑动窗口操作
[12]:
da_smooth = da.rolling(time=30).mean().persist()
da_smooth
[12]:
<xarray.DataArray 'air' (time: 2920, lat: 25, lon: 53)> dask.array<truediv, shape=(2920, 25, 53), dtype=float64, chunksize=(2920, 25, 25), chunktype=numpy.ndarray> Coordinates: * lat (lat) float32 75.0 72.5 70.0 67.5 65.0 ... 25.0 22.5 20.0 17.5 15.0 * lon (lon) float32 200.0 202.5 205.0 207.5 ... 322.5 325.0 327.5 330.0 * time (time) datetime64[ns] 2013-01-01 ... 2014-12-31T18:00:00 Attributes: long_name: 4xDaily Air temperature at sigma level 995 units: degK precision: 2 GRIB_id: 11 GRIB_name: TMP var_desc: Air temperature dataset: NMC Reanalysis level_desc: Surface statistic: Individual Obs parent_stat: Other actual_range: [185.16 322.1 ]
由于 xarray 将其每个坐标变量存储在内存中,因此按标签进行切片非常简单且完全是延迟计算的。
[13]:
%time da.sel(time='2013-01-01T18:00:00')
CPU times: user 1.05 ms, sys: 2.82 ms, total: 3.87 ms
Wall time: 7.08 ms
[13]:
<xarray.DataArray 'air' (lat: 25, lon: 53)> dask.array<getitem, shape=(25, 53), dtype=float32, chunksize=(25, 25), chunktype=numpy.ndarray> Coordinates: * lat (lat) float32 75.0 72.5 70.0 67.5 65.0 ... 25.0 22.5 20.0 17.5 15.0 * lon (lon) float32 200.0 202.5 205.0 207.5 ... 322.5 325.0 327.5 330.0 time datetime64[ns] 2013-01-01T18:00:00 Attributes: long_name: 4xDaily Air temperature at sigma level 995 units: degK precision: 2 GRIB_id: 11 GRIB_name: TMP var_desc: Air temperature dataset: NMC Reanalysis level_desc: Surface statistic: Individual Obs parent_stat: Other actual_range: [185.16 322.1 ]
[14]:
%time da.sel(time='2013-01-01T18:00:00').load()
CPU times: user 23.5 ms, sys: 7.2 ms, total: 30.7 ms
Wall time: 91.1 ms
[14]:
<xarray.DataArray 'air' (lat: 25, lon: 53)> array([[241.89 , 241.79999, 241.79999, ..., 234.39 , 235.5 , 237.59999], [246.29999, 245.29999, 244.2 , ..., 230.89 , 231.5 , 234.5 ], [256.6 , 254.7 , 252.09999, ..., 230.7 , 231.79999, 236.09999], ..., [296.6 , 296.4 , 296. , ..., 296.5 , 295.79 , 295.29 ], [297. , 297.5 , 297.1 , ..., 296.79 , 296.6 , 296.29 ], [297.5 , 297.69998, 297.5 , ..., 297.79 , 298. , 297.9 ]], dtype=float32) Coordinates: * lat (lat) float32 75.0 72.5 70.0 67.5 65.0 ... 25.0 22.5 20.0 17.5 15.0 * lon (lon) float32 200.0 202.5 205.0 207.5 ... 322.5 325.0 327.5 330.0 time datetime64[ns] 2013-01-01T18:00:00 Attributes: long_name: 4xDaily Air temperature at sigma level 995 units: degK precision: 2 GRIB_id: 11 GRIB_name: TMP var_desc: Air temperature dataset: NMC Reanalysis level_desc: Surface statistic: Individual Obs parent_stat: Other actual_range: [185.16 322.1 ]
自定义工作流程和自动并行化¶
几乎所有 xarray 的内置操作都适用于 Dask 数组。如果您想使用一个不是由 xarray 包装的函数,一个选项是从 xarray 对象 (.data
) 中提取 Dask 数组并直接使用 Dask。
另一个选项是使用 xarray 的 apply_ufunc()
函数,它可以自动执行易于并行化的“映射”类型操作,其中为处理 NumPy 数组而编写的函数应重复应用于包含 Dask 数组的 xarray 对象。它的工作方式类似于 dask.array.map_blocks()
和 dask.array.blockwise()
,但无需中间抽象层。
在这里,我们展示一个使用 NumPy 操作和 bottleneck
中的一个快速函数的示例,我们用它来计算 Spearman 秩相关系数
[15]:
import numpy as np
import xarray as xr
import bottleneck
def covariance_gufunc(x, y):
return ((x - x.mean(axis=-1, keepdims=True))
* (y - y.mean(axis=-1, keepdims=True))).mean(axis=-1)
def pearson_correlation_gufunc(x, y):
return covariance_gufunc(x, y) / (x.std(axis=-1) * y.std(axis=-1))
def spearman_correlation_gufunc(x, y):
x_ranks = bottleneck.rankdata(x, axis=-1)
y_ranks = bottleneck.rankdata(y, axis=-1)
return pearson_correlation_gufunc(x_ranks, y_ranks)
def spearman_correlation(x, y, dim):
return xr.apply_ufunc(
spearman_correlation_gufunc, x, y,
input_core_dims=[[dim], [dim]],
dask='parallelized',
output_dtypes=[float])
在上面的示例中,我们使用了气温数据。对于此示例,我们将使用原始气温数据与我们创建的平滑版本 (da_smooth
) 来计算 spearman 相关性。为此,我们还需要提前对数据进行重新分块。
[16]:
corr = spearman_correlation(da.chunk({'time': -1}),
da_smooth.chunk({'time': -1}),
'time')
corr
[16]:
<xarray.DataArray 'air' (lat: 25, lon: 53)> dask.array<transpose, shape=(25, 53), dtype=float64, chunksize=(25, 25), chunktype=numpy.ndarray> Coordinates: * lat (lat) float32 75.0 72.5 70.0 67.5 65.0 ... 25.0 22.5 20.0 17.5 15.0 * lon (lon) float32 200.0 202.5 205.0 207.5 ... 322.5 325.0 327.5 330.0
[17]:
corr.plot(figsize=(12, 8))
[17]:
<matplotlib.collections.QuadMesh at 0x7fc70cd2fdc0>
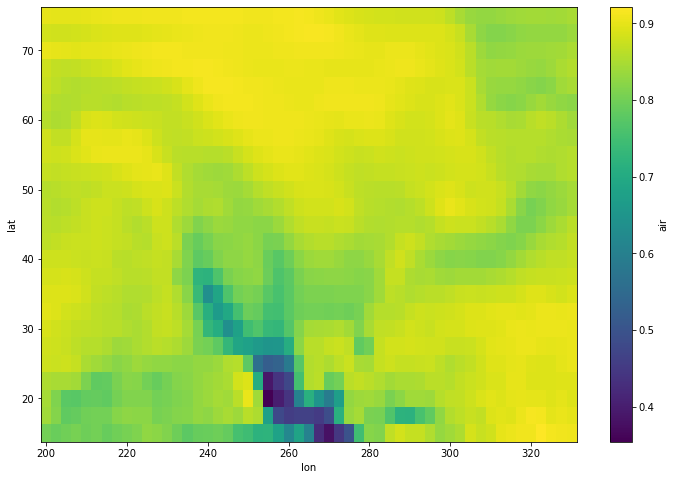